Table of Contents
In the rapidly evolving world of artificial intelligence (AI), two fascinating concepts have been making waves: Multimodal AI and Generative AI. Both are at the forefront of technological innovation, pushing the boundaries of what machines can do. If you’re an information technology engineer, understanding the nuances between these two is crucial, as it can significantly impact your projects and career trajectory.
What is Multimodal AI?
Multimodal AI is an advanced form of AI that can process and integrate multiple types of data simultaneously. This means it can understand and analyze data from various modalities like text, images, audio, and even video. The goal is to create a more holistic understanding by leveraging the strengths of each data type.
Real-Life Example: Self-Driving Cars
Consider self-driving cars, a prime example of multimodal AI in action. These vehicles use cameras (vision), LIDAR (depth perception), and microphones (sound) to navigate roads safely. By integrating these different data types, the AI can make informed decisions, like recognizing a pedestrian crossing the street or hearing a siren from an emergency vehicle.
What is Generative AI?
Generative AI, on the other hand, focuses on creating new content. It uses existing data to generate new, previously unseen data. This can include creating realistic images, writing text, composing music, and even coding. Generative AI models learn the underlying patterns in the training data and use this knowledge to produce new instances that are similar yet unique.
Real-Life Example: ChatGPT
A common example of generative AI is language models like ChatGPT. These models can generate coherent and contextually relevant text, making them useful for applications like customer service chatbots, content creation, and even code generation. They can also generate new images, like those you might see in AI-generated art.
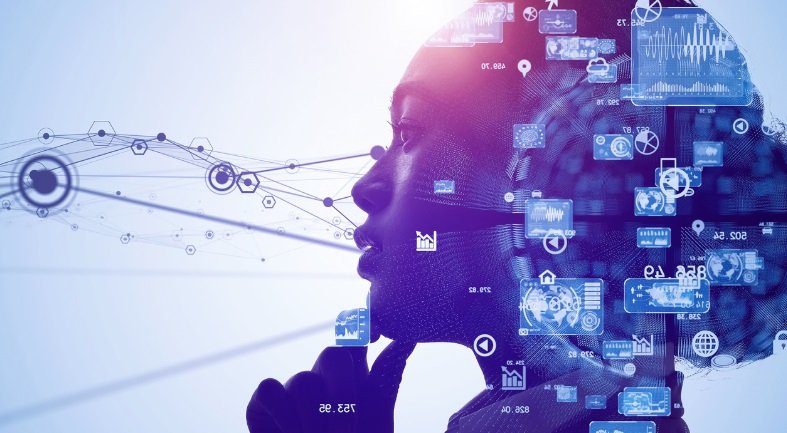
Key Differences and Similarities
While both multimodal AI and generative AI are subsets of artificial intelligence, they serve different purposes:
- Data Types: Multimodal AI deals with multiple data types, while generative AI usually focuses on creating new data within a specific domain.
- Applications: Multimodal AI is often used in scenarios requiring the integration of diverse data, such as autonomous vehicles or healthcare diagnostics. Generative AI excels in creative tasks, like generating art, music, or even new scientific hypotheses.
- Complexity: Multimodal AI is often more complex to implement because it involves integrating various types of data, each with its own processing requirements. Generative AI, while also complex, primarily focuses on mastering and creating within a single data type or domain.
The Convergence: When Multimodal and Generative AI Meet
Interestingly, these two technologies are not mutually exclusive. There is a growing trend toward developing AI systems that can both integrate multiple data types and generate new content. For instance, OpenAI’s DALL-E can create images from textual descriptions, combining the capabilities of multimodal understanding with generative creativity.
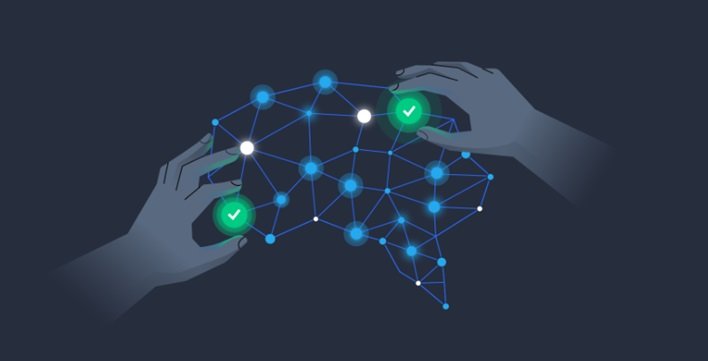
Challenges and Future Directions
Both multimodal AI and generative AI come with challenges. For multimodal AI, the primary issue is data alignment—how to ensure that information from different modalities is synchronized and accurately interpreted. For generative AI, the challenge lies in ensuring the quality and ethical implications of the generated content. For example, deepfakes, which use generative models, can be used for malicious purposes.
As these technologies evolve, they will likely become more intertwined, leading to even more sophisticated AI systems. For information technology engineers, staying updated with these advancements is not just a career advantage but a necessity.
Conclusion
In the debate between multimodal AI and generative AI, there is no clear winner. Each serves its unique purpose and has its own set of challenges and opportunities. Understanding these technologies, their applications, and their future directions will enable you to leverage them effectively in your projects. Whether you’re developing a new AI system or integrating AI into existing solutions, knowledge of both multimodal and generative AI will be invaluable.
As we move forward, the line between these two types of AI may blur, giving rise to more versatile and powerful systems. So, buckle up and stay tuned for the exciting developments ahead!
Leave a Reply