Table of Contents
Introduction – Data Analytics and Machine Learning
Choosing the right data analytics and machine learning platform can be a game-changer for your business. But with so many options out there, it can be overwhelming. Let’s break down the key factors to consider, and I’ll share some real-life examples to make things clearer.
1. Define Your Goals and Use Cases
First, identify what you want to achieve with data analytics and machine learning (ML). Are you looking to improve customer experience, optimize operations, or predict market trends? Each goal may require different capabilities from your platform.
Example: A retail company aiming to enhance customer experience might prioritize platforms with strong customer segmentation and recommendation engines. On the other hand, a manufacturing firm focused on predictive maintenance would look for robust anomaly detection and forecasting features.
2. Ease of Use and Accessibility
Consider who will be using the platform. Is it designed for data scientists, business analysts, or a mix of both? A user-friendly interface can make a huge difference in adoption rates and productivity.
Example: Tableau is known for its user-friendly drag-and-drop interface, making it accessible for business users without a technical background. Meanwhile, platforms like Apache Spark are preferred by data scientists for their powerful processing capabilities but may have a steeper learning curve.
3. Integration Capabilities
Ensure the platform integrates well with your existing tools and systems. Seamless integration can save time and reduce complexity in your workflows.
Example: A company using Salesforce for customer relationship management (CRM) would benefit from a platform like Tableau, which offers native integration with Salesforce, enabling easy data sharing and insights generation.
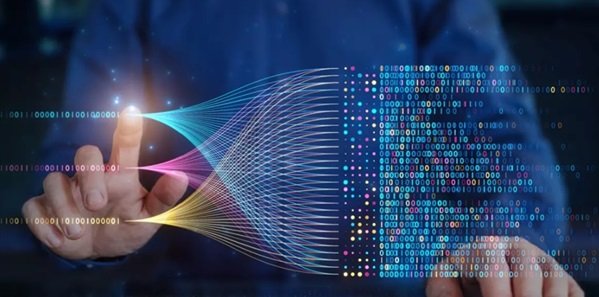
4. Scalability and Performance
Your platform should be able to handle your data volume and scale as your needs grow. Performance is critical, especially when dealing with large datasets and real-time analytics.
Example: Netflix relies on Apache Kafka for real-time data streaming and Apache Flink for processing large-scale data streams. This combination allows Netflix to deliver personalized content recommendations to millions of users in real-time.
5. Advanced Analytics and Machine Learning Capabilities
Look for platforms that offer advanced analytics and ML features, such as predictive modeling, natural language processing (NLP), and deep learning.
Example: Google Cloud AI Platform provides a comprehensive suite of ML tools, including AutoML for building custom models with minimal coding, and TensorFlow for advanced deep learning applications. This versatility can cater to both beginners and experts in ML.
6. Security and Compliance
Data security and compliance with regulations (like GDPR) are non-negotiable. Ensure the platform provides robust security features and complies with relevant regulations.
Example: Healthcare companies dealing with sensitive patient data must comply with HIPAA regulations. Platforms like Microsoft Azure offer compliance with various standards, including HIPAA, ensuring that data is secure and regulations are met.
7. Cost and ROI
Consider the cost of the platform and the return on investment (ROI) it promises. Evaluate the pricing model—whether it’s subscription-based, pay-as-you-go, or based on data volume.
Example: Startups might opt for cost-effective solutions like AWS Free Tier, which offers a range of free services for a limited time, allowing them to scale without significant upfront investment. In contrast, large enterprises might invest in comprehensive platforms like SAS Analytics for their robust features and enterprise-level support.
8. Support and Community
Good customer support and an active user community can help you troubleshoot issues and get the most out of your platform.
Example: Platforms like AWS and Google Cloud have extensive documentation, active forums, and dedicated support teams. Additionally, community platforms like Stack Overflow provide a wealth of knowledge and peer support.
Conclusion – Data Analytics and Machine Learning
Choosing the right data analytics and machine learning platform involves balancing multiple factors based on your specific needs and goals. By considering aspects like ease of use, integration capabilities, scalability, advanced analytics features, security, cost, and support, you can find a platform that not only meets your current requirements but also grows with your business.
Remember, the best platform is one that aligns with your strategic objectives and empowers your team to turn data into actionable insights. Take your time to evaluate options, perhaps even trial a few, and choose a platform that will drive your data-driven success.
1 Pingback